Key Role of NLP in Electronic Health Records
- January 8, 2024
- Posted by: Chaitali Avadhani
- Category: Healthcare Technology
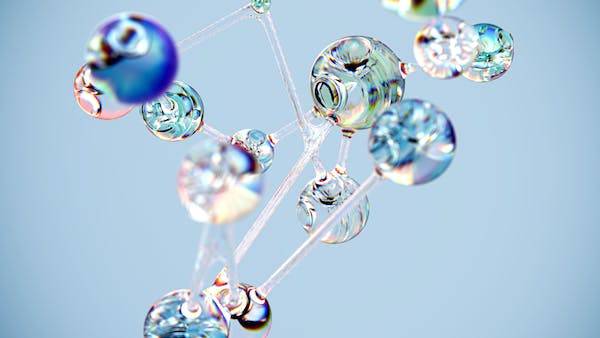
Key Takeaways
- Capabilities of NLP to summarize notes and categorize medical data can simplify or rather automate the documentation process.
- NLP in electronic health records identifies key terms from the unstructured notes and extracts them for further use which is clinical decision-making.
- The clinical data contains information on patient symptoms, treatment plans, and diagnosis and it is analyzed by providers to gain insights.
When it comes to data in healthcare, EHR is the go-to option. However, a certain time is required to document patient data and extract it when needed. Reports suggest that healthcare providers spend 3.5 hours on clinical documentation alone. The root cause of physician burnout is prolonged periods of clinical documentation.
Natural language processing (NLP) is a popular branch of AI that’s used for speech recognition, text extraction, and conversion of unstructured data in healthcare. When used in EHR, NLP can automate not only clinical documentation, but also aid in healthcare decision-making, and improve the standard of care.
This article highlights how NLP plays a key role in electronic health records.
Role of NLP in Electronic Health Records
1. NLP for Clinical Documentation
Healthcare providers are confronted with a need for high-quality and timely patient documentation. Low physician satisfaction and time pressure are associated with increased clinical documentation.
Right from compiling consultation notes to offering e-prescriptions, everything goes into the EHR, and if failed to do so on time can have adverse effects. For instance, if a patient calls in for chest pain and the physician advises to go to the ER, but the patient doesn’t go and faces cardiac arrest later. He may sue the physician for not sending him to the ER, but if the doctor has documented every treatment step, then can be saved from court.
NLP in electronic health records can make documentation tasks easier for healthcare providers. The capabilities of NLP to summarize notes and categorize medical data can simplify or rather automate the documentation process. Recently, AWS announced the launch of AWS HealthScribe, a HIPAA service. It uses speech recognition and generative AI to save physicians’ time in clinical documentation.
Another development in the field is the partnership between HCA Healthcare and Augmedix which is aimed at augmenting the development of AI-enabled ambient documentation solutions for acute care providers. The solution uses NLP and automatic speech recognition to convert provider-patient interactions to medical notes. The notes are finalized by the care team before adding them to the EHR. The focus is to reduce clinical burnout and prioritize EHR optimization.
2. NLP for Unstructured Data
Unstructured data is not in a readable format, hence it needs to be converted into a structured format. Healthcare organizations must leverage technology such as NLP to convert unstructured data into a structured format.
Natural language processing identifies keywords in the data and derives meaning from it. For instance, a provider’s note on dietary counseling may read as the patient enrolled for obesity along with the patient’s treatment plan. In the case of a smoker, the provider might document ‘chain smoker’ or ‘smokes twice a day.’
NLP in electronic health records identifies key terms from the unstructured notes such as ‘smoker or not a smoker’ and extracts it for further use which is clinical decision-making. The majority of the information may be lost in unstructured data such as the sugar levels of a smoker, but NLP ensures it captures essential information and brings it to the table.
An example would be Melax Tech, an AI-driven software provider of NLP technology, who introduced the launch of Mercury NLP. This software offers clinical NLP pipelines to extract unstructured textual medical data for analysis in the pharmaceutical and medical fields.
In 2023, Concensus Cloud Solutions, Inc., the largest digital cloud fax and interoperability solutions announced the launch of Clarity Clinical Documentation. This is a cost-effective solution that automatically routes unstructured data from clinical data faxes, scanned documents, and handwritten notes into patient records.
With the help of NLP and AI, Cloud Clarity extracts key patient demographics from unstructured data into structured CCD (Continuity of Care Document), and then the information is sent to the EHR.
3. For Clinical Decision-Making
Electronic health records are a storehouse of patient data that can be summarized, extracted, and analyzed for clinical decision-making. Keyword extraction, pre-processing, named entity recognition (NER), and text summarization are some techniques used by natural language processing for extracting information from the EHR. This information contains data on patient symptoms, treatment plans, diagnosis, and recommendations from medical notes which are analyzed to gain insights.
NLP in electronic health records helps physicians in clinical decision-making based on insights from medical texts. It automatically systematically arranges crucial information. These insights improve overall care and patient outcomes.
A study on AI by JAMA Cardiology examined the potential for natural language processing (NLP) models to accurately classify heart failure (HF) hospitalizations compared with clinical adjudication.
Future of NLP in Electronic Health Records
The future of NLP in electronic health records is bright because NLP is a rapidly advancing technology with the ability to transform the way healthcare is delivered in today’s time.
From the article, it’s clear that natural language processing is used to improve the efficacy of clinical documentation and healthcare delivery. For instance, NLP can automate tasks such as summarizing notes, extracting information, and converting unstructured data into a structured format. This frees up healthcare practitioners to spend more time with patients.
Uses of NLP in healthcare is still in the early stages. With the introduction of generative AI, NLP capabilities have gone up and will continue to grow. In the future, NLP in electronic health records can be used to reduce healthcare costs, improve patient safety, or bolster quality of care.
As we witness the evolution of healthcare through NLP, it is imperative to embrace its potential responsibly, ensuring ethical use and patient privacy. The journey towards a more efficient, personalized, and accessible healthcare future is underway.
If you’re looking for AI technology for your organization, then connect with Arkenea, a leading healthcare software development company in the USA. We’ve over 13 years of experience in the field and our team of expert AI developers delivers products based on your requirements that match industry standards.