Top 6 Machine Learning Applications in Healthcare
- March 29, 2023
- Posted by: Chaitali Avadhani
- Category: Healthcare Technology
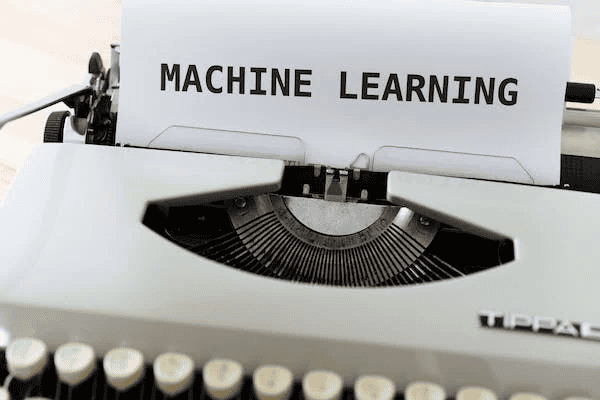
Key Takeaways
- According to a report published in the JAMA Network Open in 2021, machine learning models can be used to predict COVID-19 clinical severity with the help of large-scale US COVID-19 data resources.
- One of the key machine learning applications in healthcare is clinical trial and research. ML helps to automate surveys, capture voice clinical data, and analyze data in less time.
- As per an article machine learning and deep learning methods such as CNNs (Convolutional Neural Networks) and RNNs (Recurrent Neural Networks) can maximize information output from neurological images.
Machine learning, a branch of artificial intelligence can replicate the thinking process of a human mind. It answers our queries, solves complex mathematical equations, and automates cumbersome tasks. It has spread across multiple sectors because of these abilities, healthcare being one of them.
Healthcare AI development companies are heavily innovating and investing in the research and development of machine learning applications. Some of these applications are described in this blog article.
6 Machine Learning Applications in Healthcare
1. Predictive Analytics
Predictive analytics has changed the way doctors treat and diagnose patients. Machine learning algorithms are programmed to provide insights into patients’ medical data. Physicians use these insights to predict diagnosis and treatment plans, which eventually improves patient outcomes.
a. Infectious Disease Outbreak Prediction
Infectious diseases such as the recent COVID-19 virus have caused grave damage to not only the human body but also the economy. Hence, it’s necessary to keep tabs on emerging infectious diseases in the upcoming years. This can be achieved by using machine learning tools.
According to a report published in the JAMA Network Open in 2021, machine learning models can be used to predict COVID-19 clinical severity with the help of large-scale US COVID-19 data resources.
Apart from this, another machine learning application in healthcare includes early or real-time detection of epidemics. For instance, a research paper published on mdpi.com in 2023 stated that with multiple ML models, it is possible to spatially and temporally predict trends of infectious diseases.
b. Identifying Disease and Diagnosis
Healthcare providers use machine learning to predict diseases, especially chronic illnesses. With predictive analytics, they can intervene and prevent future cases of chronic diseases, and treat those who show initial symptoms.
Diseases are detected by scanning through EHR data. The researchers at Michigan State University are developing an AI app that uses predictive analytics to determine whether a person is showing signs of Alzheimer’s disease.
c. Predictive Treatment
Once the right disease is identified, doctors can again turn to machine learning to estimate the right treatment options. According to a study published on the JAMA Network in 2023, a machine learning predictive model can successfully be used for treatment allocation for recurrent hepatocellular carcinoma (HCC) post-surgery.
Predictive treatment is only possible after feeding the right data to an ML algorithm and scanning through countless medical records.
2. Drug Discovery and Production
The next machine learning application in healthcare is a drug discovery and production. Here, ML can predict the target drug and model structure for this targeted drug. It is also used for estimating the binding site of a drug, designing ligands with desired properties, and molecular docking.
Researchers from Carnegie Mellon University have developed a self-supervised learning framework to predict molecular properties. The framework is called MolCLR and it boosts the performance of ML models by leveraging around 10 million unlabeled molecular data. It can be used in the drug production process.
3. Clinical Trial and Research
Machine learning holds great promise for improving the efficiency of clinical trials and research. It helps to automate surveys, capture voice clinical data, and analyze data in less time. Additionally, ChatGPT in healthcare, a generative AI, is used for research and manuscript writing. Researchers can avail of this tool to write their thesis, and research papers, and to discover new clinical research ideas.
ML has its own set of barriers in clinical research. For instance, a research paper published on the JAMA Network stated that there are concerns regarding the quality of medical machine learning RCTs (Randomized Clinical Trials). It points out suggestions to improve inclusivity and reporting transparency for the publication of future clinical trials.
Based on the data gathered, machine learning algorithms estimate clinical trial participants’ dropout risks. So, researchers can take early steps to prevent it and continue with the trials. Data analyses with machine learning help with clinical decision support too. Researchers can speculate about trial stages and potential issues associated with them.
4. Personalized Medicine
Personalized medicine or precision medicine is a tailored approach to disease prevention and treatment. It takes into account patients’ lifestyles, genetics, and environment. Healthcare providers can use the approach of personalized medicine after collecting data from patients. This data is acquired via wearables, medical devices, and varied healthcare software used by patients.
The application of machine learning in precision medicine is to scrutinize this data. Once data is analyzed, providers can prepare a personal treatment plan, based on the insights gathered from the healthcare data.
5. Medical Imaging Diagnosis
Diseases such as Alzheimer’s, Parkinson’s, cancer, and any other neurodegenerative diseases can be best diagnosed with images. Modalities used to capture images of patients suffering from these types of diseases include MRI, SPECT, and PET. However, analyzing these images is a cumbersome task as they’re hardly brought to their full potential. This is a major obstacle for medical image diagnosis procedures. It can be overcome with deep learning or machine learning technology and the same is being leveraged by healthcare AI developers.
An article published in the Physics World stated that deep learning methods such as CNNs (Convolutional Neural Networks) and RNNs (Recurrent Neural Networks) can maximize information output from neurological images. It can augment the understanding of neurodegenerative diseases and help in clinical decision making too.
6. Robotic Surgery
Another machine learning application in healthcare includes robotic surgery. ML contributes towards suturing, a process of sewing up an incision or an open wound. Suturing is a time-consuming process and ML-driven automation reduces the length of the procedure.
Further, ML is used to evaluate surgical skills. Algorithms are designed to asses skills across domains such as smoothness, curvature, completion time, depth perception, and path length. According to a study published by the JAMA Network in 2020, the use of robotic surgery for all general surgeries increased from 1.8 percent to 15.1 percent. High-scale use of robotic surgery saw a decrease in traditional laparoscopic minimally invasive surgery.
Apart from this, robots lack the necessary skills to operate in sensitive neurosurgery cases. So, researchers at the University of California, San Diego (UCSD) are exploring machine learning applications to ameliorate surgical robots for such operations.
Machine learning and AI will significantly grow in the upcoming years, owing to their ability to automate tasks, predict diagnosis and treatment, and assist in robotic surgery. Patients are also likely to approach facilities that provide ML and AI-based treatment options. So, get AI technology for your healthcare organization and offer best-in-class treatment to your patients.
Arkenea, a healthcare software development company provides AI powered applications for healthcare practices. It has a proven track record of delivering top-notch AI software to its clients. Connect with us to know more.